Building a cloud analytics data warehouse 101: Google Analytics 4 & Looker Studio
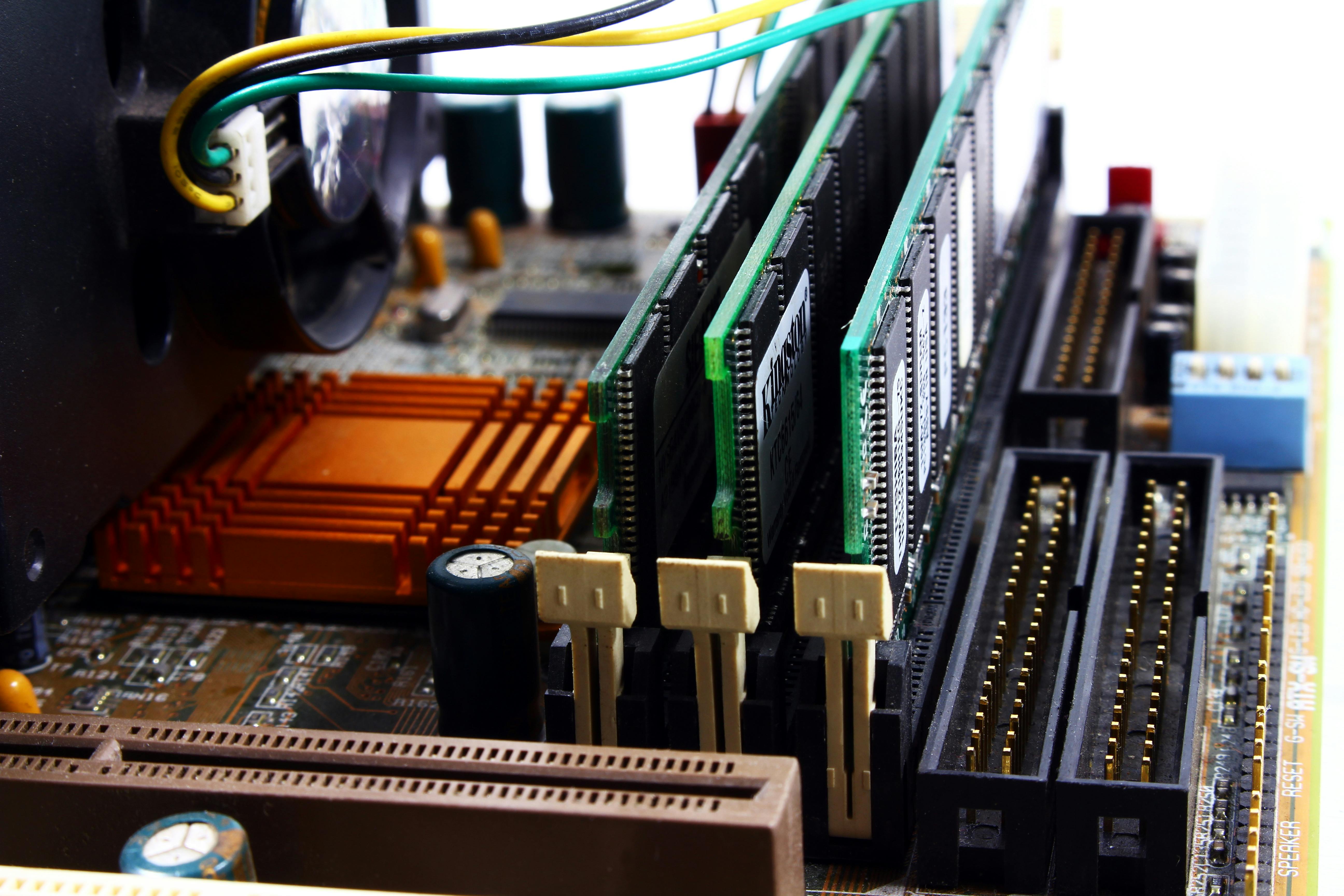
Unlock the benefits of migrating to GA4 and overcome transition challenges. Learn how GA4 export to BigQuery provides a reliable data source for Looker Studio. Discover the power of a Cloud Analytics Data Warehouse with BigQuery on Google Cloud Platform. Optimize your data analytics strategy with insights on designing structures, cost control, data integration, transformation, and security.
Universal Analytics is dead; Long live GA4. Is your business ready to move on?
Plenty of companies are well aware of the upcoming move from UA to GA4, but many do not fully understand the changes to business processes this will inevitably bring. As the sunset date of UA (Universal Analytics) approaches, many companies are transitioning to GA4 (Google Analytics 4). During our experience with client transitions, two key points became evident:
Technical Switch: Moving from UA to GA4 is relatively straightforward from a technical standpoint. It also provides an opportunity to revisit your data collection requirements and clean up any outdated practices.
Business Transition: However, the business aspect of the switch can be more challenging. Teams are often reluctant to adopt a new system, and clients may not fully realize the extent to which their UA data is used across various endpoints. The main challenge that arises is with dashboards.
Problem: Reluctance to switch. Overlooked endpoints. Dashboard issues.
From a technical perspective, switching between UA and GA4 is relatively easy. However, the business transition can be complicated. Teams may resist switching to a new system, and clients may not anticipate the number of endpoints that rely on UA data. The primary obstacle is often encountered when connecting GA4 data to Google Looker (previously known as Data Studio), as Google's introduction of API limits renders many dashboards ineffective.
'Google's introduction of API limits renders many dashboards ineffective.'
Solution: Leveraging GA4 Export to BigQuery
Fortunately, Google has increased flexibility in the native connection between Looker and GA4 for companies with 360 accounts. However, if you don't have a 360 account, need access to specific data points not yet available in GA4's reporting API, or simply want to avoid rate limits altogether, the direct Looker connection may not be suitable. In such cases, the most practical solution is to enable GA4 export to BigQuery and utilize it as a data source for Google Looker Studio. With just a few simple steps, raw data can be automatically imported on a daily basis, ensuring your dashboards have all the necessary information to operate seamlessly.
One Small Step, One Massive Leap for Your Data
Enabling GA4 export may seem like a small step, but it has significant implications beyond maintaining Looker dashboards. By taking this action, you embark on a journey into a Cloud Analytics Data Warehouse and unlock numerous potential benefits. You gain access to a comprehensive cloud project that can cater to a wide range of data use cases. In the upcoming parts of this series, we will explore specific use cases that can be achieved using a Cloud Analytics Data Warehouse.
Why Choose a Cloud Analytics Data Warehouse?
A Cloud Analytics Data Warehouse, such as BigQuery, is a fully managed environment provided by the Google Cloud Platform. It eliminates the need for setting up, maintaining, and scaling underlying servers, as Google handles these aspects for you. The primary task for users is to integrate all relevant data into the BigQuery environment, opening the door to valuable insights. Since BigQuery efficiently scales server power, it allows for querying vast amounts of data without the need for complex optimizations. Additionally, BigQuery seamlessly integrates with various data visualization tools, empowering users to create informative dashboards. Furthermore, integrating other data sources into your Cloud Data Warehouse enhances its capabilities. By combining analytics data with online marketing data, product information, or return data, you can compare web behavior, report on specific categories or products, and identify patterns. The Cloud Analytics Data Warehouse provides the foundation for these advanced analytics use cases.
Why Choose a Cloud Environment over Current Database Providers?
The decision to adopt a Cloud Analytics Data Warehouse over existing database providers, such as SAP or Azure, depends on organizational factors. While consolidating all data in one place may seem appealing, it can limit operational agility in practice. Existing data warehouse environments often prioritize data safety and may not facilitate quick insights and integrations. Integrating raw analytics data into these environments can be challenging.
Performance Considerations and the Importance of an Entire Cloud Environment
Another critical factor to consider is performance. While most modern environments can handle advanced queries on raw analytics data, existing Data Warehouses are often not designed to handle such large volumes of data efficiently. Enhancing the current environment to accommodate raw analytics data may result in significant additional costs that may not always justify the value it brings. Moreover, traditional data warehouses are primarily geared towards working with factual data, such as finance reporting, where the emphasis is on generating accurate numbers. In contrast, a Cloud Analytics Data Warehouse focuses on identifying patterns and explains relationships in data. The existing tooling and users may not be equipped to handle these types of analytical use cases effectively. Whether these considerations apply to your organization depends on your specific circumstances. It is advisable to make a deliberate decision about splitting Data Warehouse activities into two environments, with agreement from both Data Warehouse owners, rather than simply creating a secondary location for data without broader awareness. Additionally, it is crucial to define the boundaries for building and maintaining data in each environment.
Why an Entire Cloud Environment Matters
While BigQuery alone offers numerous possibilities, the additional advantages of accessing the entire Google Cloud Platform (GCP) should not be overlooked. GCP provides a wide range of tools that facilitate the integration of external data sources, preparation of structured and unstructured data for various use cases, and backup storage in the cloud. When you're ready to explore more advanced scenarios, GCP provides a powerful interface for training and deploying machine learning models, including pre-trained models that can be utilized immediately. Furthermore, specific tooling is available to expose your APIs in a user-friendly manner when you want to share specific data with a website or app environment.
“Don't just make the switch. Flip the switch on your data processing capabilities.”
Starting Off on the Right Foot
While the new environment opens up numerous possibilities, it's essential to recognize that setting up a Data Warehouse correctly is crucial to unlocking its potential benefits. Many long-running Data Warehouses encounter issues with performance, costs, or usability simply because a clear strategy and architecture were not established from the outset. Since your implementation is still in its early stages, it is an opportune time to start off on the right track.
In the subsequent parts of this series, we will delve into important topics to consider when building your Cloud Analytics Data Warehouse. These topics include:
- Exercising control over costs by choosing appropriate pricing structures.
- Designing the structure of your datasets and tables to accommodate scalability while maintaining usability.
- Exploring methods to integrate external data sources into your environment effectively.
- Transforming your data within the Data Warehouse to ensure optimal performance and scalability.
- Building a foundation of security, privacy, and maintainability.
By addressing these aspects, you can lay the groundwork for a robust and efficient Cloud Analytics Data Warehouse that maximizes the potential benefits for your organization.
Conclusion
By leveraging GA4 and Loo ker Studio, you can embark on a transformative data analytics journey. These powerful tools serve as excellent starting points for building your own Cloud Analytics Data Warehouse. With a Cloud Analytics Data Warehouse, you gain the ability to make data-driven decisions and drive innovation within your organization. The benefits are within reach - improved data visibility, enhanced analysis, and the ability to harness the full potential of your data. The enforced move from Universal Analytics to GA4 is a blessing in disguise. Don't just make the switch, but flip the switch on your data processing capabilities. Embark on a journey into a Cloud Analytics Data Warehouse and unlock numerous potential benefits.